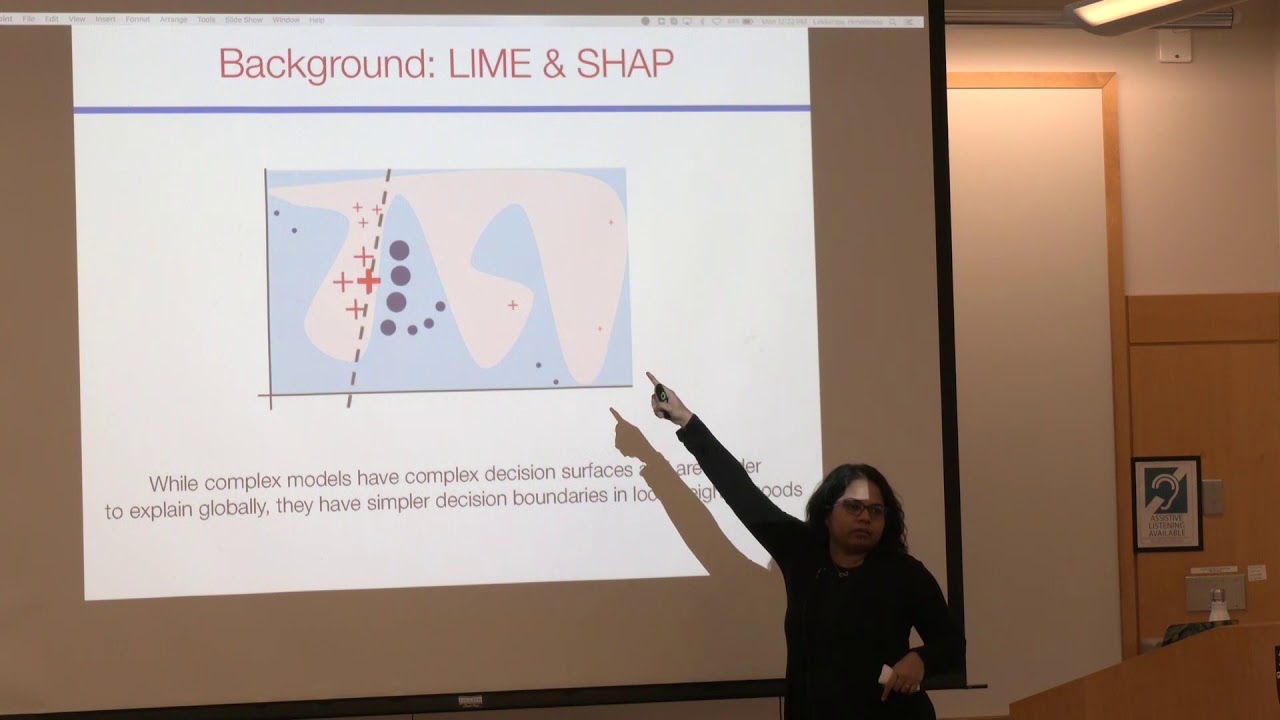
As machine learning black boxes are increasingly being deployed in domains such as healthcare and criminal justice, there is growing emphasis on building tools and techniques for explaining these black boxes in an interpretable manner. Such explanations are being leveraged by domain experts to diagnose systematic errors and underlying biases of black boxes. In this talk, I will demonstrate that post hoc explanations techniques that rely on input perturbations, such as LIME and SHAP, are not reliable. Specifically, I will discuss a novel scaffolding technique that effectively hides the biases of any given classifier by allowing an adversarial entity to craft an arbitrary desired explanation. Our approach can be used to scaffold any biased classifier in such a way that its predictions on the input data distribution still remain biased, but the post hoc explanations of the scaffolded classifier look innocuous. Using results from real world datasets (including COMPAS), I will demonstrate how extremely biased (racist) classifiers crafted by our framework can easily fool popular explanation techniques such as LIME and SHAP into generating innocuous explanations which do not reflect the underlying biases. I will conclude the talk by discussing some user studies that we carried out to understand the perils of such misleading explanations and how they can be used to manipulate user trust.
Hima Lakkaraju will be starting as an Assistant Professor at Harvard University in January 2020. She is currently a postdoctoral fellow at Harvard and has recently graduated with a PhD in Computer Science from Stanford University. Her research focuses on building accurate, interpretable, and fair AI models which can assist decisions makers (e.g., judges, doctors) in critical decisions (e.g., bail decisions). Her work finds applications in high-stakes settings such as criminal justice, healthcare, public policy, and education. At the core of her research lie rigorous computational techniques spanning AI, ML, and econometrics. Hima has recently been named one of the 35 innovators under 35 by MIT Tech Review, one of the innovators to watch by Vanity Fair, and has received several fellowships and awards including the Robert Bosch Stanford graduate fellowship, Microsoft research dissertation grant, Google Anita Borg scholarship, IBM eminence and excellence award, and best paper awards at SIAM International Conference on Data Mining (SDM) and INFORMS.
0 Comments